The Ultimate Guide to Data Labelling Tools
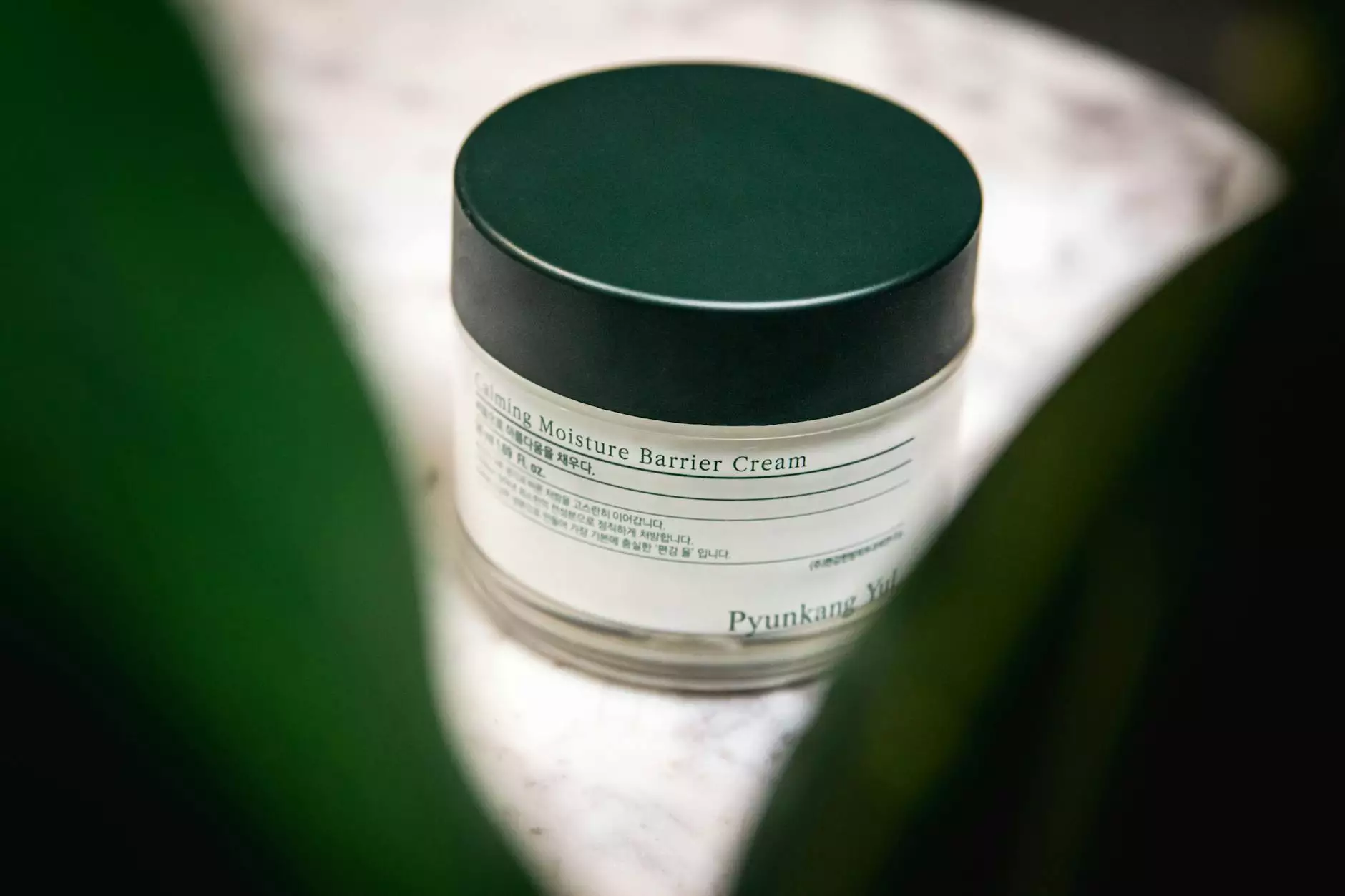
In the modern world of machine learning and artificial intelligence, the importance of data labelling tools cannot be overstated. These tools are not just a component of data processing; they form the backbone of any data-driven decision-making process. In this article, we will explore the significance of data labelling, the features that make a data labelling tool effective, and how businesses can leverage these tools to improve their operations.
What is a Data Labelling Tool?
A data labelling tool is a software application designed to assist users in annotating and labelling data, which is essential for training machine learning models. This process involves tagging different elements within a dataset, whether they be images, text, or audio. By providing a systematic way to associate labels with raw data, these tools facilitate the creation of high-quality datasets necessary for machine learning and AI initiatives.
The Importance of Data Labelling
Data labelling is crucial because it directly impacts the performance of machine learning models. Well-labelled data enhances the algorithms' ability to learn patterns, leading to more accurate predictions and insights. Understanding the various applications of data labelling is essential for businesses looking to harness AI effectively.
Applications of Data Labelling in Different Industries
- Healthcare: In healthcare, data labelling tools are used to categorize medical images, making it easier for AI systems to detect diseases accurately.
- Finance: Financial institutions use data labelling to identify fraudulent transactions and assess credit risk through data mining.
- Retail: In retail, data labelling helps in understanding customer behavior and preferences, aiding in the personalization of marketing strategies.
- Automotive: In autonomous driving, data labelling is vital for interpreting images and sensor data, allowing vehicles to navigate safely.
- Natural Language Processing: Data labelling tools help in sentiment analysis and language translation by annotating text data effectively.
Key Features of an Effective Data Labelling Tool
When selecting a data labelling tool, it is essential to consider its features. The right tool should not only facilitate easy labelling but also support various types of data. Here are some significant features to look for:
1. User-Friendly Interface
A clean and intuitive interface allows users to navigate the data labelling process efficiently. This reduces the training time required for new users and enhances productivity.
2. Support for Multiple Data Formats
The best data labelling tools support various data types, including images, text, audio, and video. This versatility allows businesses to use one tool for all their labelling needs.
3. Collaboration Features
Robust collaboration features enable teams to work together seamlessly. Users should be able to assign tasks, review labelled data, and provide feedback within the platform.
4. Integrations with Machine Learning Frameworks
Integration capabilities with popular machine learning frameworks like TensorFlow and PyTorch can streamline the workflow, allowing for easier export of labelled data.
5. Automation and AI Assistance
Some advanced data labelling tools incorporate AI to assist in the labelling process, significantly speeding up the workflow and reducing human error.
How to Choose the Right Data Labelling Tool for Your Business
Choosing the right data labelling tool involves several considerations. Here’s a step-by-step guide to help you make an informed decision:
Step 1: Define Your Project Requirements
Understanding your data labelling requirements is essential. Identify the type of data you will be labelling, the size of your dataset, and the specific objectives of your project.
Step 2: Evaluate Available Tools
Research and compile a list of available data labelling tools. Look for user reviews, case studies, and feature comparisons to narrow down your options.
Step 3: Request Demos and Trials
Once you have shortlisted a few tools, request demos or sign up for free trials. This hands-on experience will give you insight into the tool’s usability and effectiveness.
Step 4: Assess Support and Resources
Evaluate the support offered by the tool provider. Access to resources such as tutorials, customer support, and community forums can significantly impact your experience.
Step 5: Consider Your Budget
Finally, consider your budget. While it's tempting to choose the cheapest option, it’s wise to weigh the cost against the features provided to find the best value for your investment.
Popular Data Labelling Tools
There are several prominent data labelling tools in the market today. Here’s a quick overview of some leading options:
- Labelbox: A powerful platform designed for image, video, and text labelling, featuring collaborative capabilities.
- SuperAnnotate: Focuses on computer vision tasks, offering an intuitive labeling platform combined with project management features.
- Amazon SageMaker Ground Truth: A cloud-based solution that harnesses Amazon’s machine learning capabilities to streamline data labelling.
- VGG Image Annotator (VIA): A lightweight and highly flexible tool for image annotation that's popular among researchers.
- Prodigy: A modern, scriptable annotation tool known for its integration with machine learning workflows.
The Future of Data Labelling
The future of data labelling tools looks promising, as advancements in AI and machine learning continue to transform the landscape. With the increasing amount of data generated daily, efficient labelling processes will become even more vital. Here are some trends to watch out for:
1. Enhanced Automation through AI
As machine learning technology evolves, automation within data labelling will become more refined, allowing for faster and more accurate labelling processes.
2. Greater Emphasis on Security and Compliance
With privacy regulations becoming stricter, data labelling tools will need to implement enhanced security measures to protect sensitive information.
3. Integration of Active Learning Techniques
Active learning allows models to identify the most informative data points for labelling, optimizing the overall labelling process.
4. Growth of Community-driven Labelling
Community-driven approaches, where large groups of users contribute to labelling efforts, may increase efficiency and data diversity.
Conclusion
The landscape of machine learning is evolving rapidly, and the demand for high-quality labelled data is at an all-time high. Leveraging a top-tier data labelling tool like those offered by Keylabs.ai is crucial for businesses aiming to stay competitive and innovative. By understanding the importance of data labelling and selecting the right tools, organizations can unlock the full potential of their data and drive their projects towards success.
Ultimately, investing in a data labelling tool is not merely an operational choice; it is a strategic one that will define the future success of your business in an increasingly data-driven world.